Visual Domain Adaptation Challenge
(VisDA-2018)
[News] [Overview] [Data] [Prizes] [Evaluation] [Rules] [FAQ] [TASK-CV Workshop] [Organizers]News
Introducing the 2018 VisDA Challenge! Stay tuned for more dates and details coming soon. This year we are using a new shiny Syn2Real dataset collected by our team.
For details about last year's challenge and winners, see the VisDA 2017 challenge page.
Click [here] to learn about our VisDA 2022 Challenge.
- Sep 24 TASK-CV workshop presentations available.
- Sep 14 Winners announcement!
- August 1 Testing data released
- May 21 Evaluation servers are open for submission
- May 16 Training and validation data released
- April 9 Registration starts
Winners of VisDA-2018 challenge
# | Team Name | Affiliation | Score |
---|---|---|---|
1 | VARMS | JD AI Research, CV Lab | 92.3 [slides] |
2 | Diggers | University of Electronic Science and Technology of China | 69.0 [slides] |
3 | THUML | Tsinghua University | 68.3 [slides] |
# | Team Name | Affiliation | Score |
---|---|---|---|
1 | VARMS | JD AI Research, CV Lab | 48.6 [slides] |
2 | GF_ColourLab_UEA | University of East Anglia, Colour Lab | 13.5 [slides] |
3 | UQ_SAS | University of Queensland, Australia | 12.1 [slides] |
Overview
We are pleased to announce the 2018 Visual Domain Adaptation (VisDA2018) Challenge! It is well known that the success of machine learning methods on visual recognition tasks is highly dependent on access to large labeled datasets. Unfortunately, performance often drops significantly when the model is presented with data from a new deployment domain which it did not see in training, a problem known as dataset shift. The VisDA challenge aims to test domain adaptation methods’ ability to transfer source knowledge and adapt it to novel target domains.
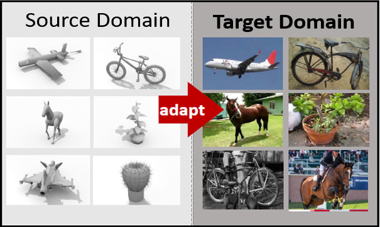
Caption: An example of a domain adaptation problem for object classification with a synthetic source (train) domain and a real target (test) domain. Unsupervised Domain Adaptation methods aim to use labeled samples from the train domain and large volumes of unlabeled samples from the test domain to reduce prediction errors on the test domain.
The competition will take place during the months of May -- September 2018, and the top performing teams will be invited to present their results at the TASK-CV workshop at ECCV 2018 in Munich, Germany. This year’s challenge focuses on synthetic-to-real visual domain shifts and includes two tracks:
across two different domain shift problems. Participants are welcome to enter in one or both tracks.
Open-Set Classification Track
Last year’s challenge featured a closed-set classification task for synthetic-to-real adaptation, where all object categories were known ahead of time. The top performing teams in this track developed CNN models that achieved impressive adaptation results. This year, we push the boundaries beyond closed-set classification and propose a novel open-set classification task. In this track, the goal is to develop a method of unsupervised domain adaptation for object classification, where the target domains contain images of additional unknown categories not present in the source dataset.
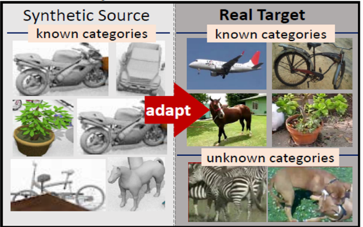
Detection Track
In this track, the goal is to develop a model that can adapt between synthetic and real objects for recognition/detection. This task entails localizing an object from each of 12 learned categories in novel images by predicting its class and its bounding box.
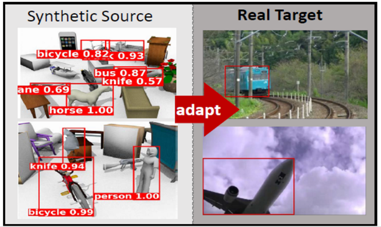
Both tracks feature the same datasets and focus on synthetic-to-real domain adaptation. Participants will be given three datasets, each containing the same object categories:
- training domain (source): synthetic 2D renderings of 3D models generated from different angles and with different lighting conditions
- validation domain (target): a photo-realistic or real-image validation domain that participants can use to evaluate performance of their domain adaptation methods
- test domain (target): a new real-image test domain, different from the validation domain and without labels . The test set will be released shortly before the end of the competition
The reason for using different target domains for validation and test is to evaluate the performance of proposed models as an out-of-the-box domain adaptation tool. This setting more closely mimics realistic deployment scenarios where the target domain is unknown at training time and discourages algorithms that are designed to handle a particular target domain.
Data
We use a recent Syn2Real dataset collected by our team. Please follow the instructions outlined in the VisDA GitHub repository to download data and development kits for the classification and segmentation tracks. Training and validation data are now available to download. We have also included baseline models and instructions on training several existing domain adaptation methods.
Prizes
We are excited to announce NVIDIA GPU prizes for the top performing teams! The top 3 winners of both the openset and detection tracks will receive:
- 1st place: Titan Xp
- 2nd place: GTX 1080 Ti
- 3rd place: GTX 1060 6GB
Evaluation
We will use CodaLab to evaluate submissions and maintain a leaderboard. To register for the evaluation server, please create an account on CodaLab and and enter as a participant in one of the following competitions:
If you are working as a team, you have the option to register for one account for your team or register multiple accounts under the same team name. If you choose to use one account, please indicate the names of all of the members on your team. This can be modified in the “User Settings” tab. If your team registers for multiple accounts, please do so using the protocol explained by CodaLab here. Regardless of whether you register for one or multiple accounts, your team must adhere to the per-team submission limits (20 entries per day per team during the validation phase).
Please refer to the instructions in the DevKit ReadMe file for specific details on submission formatting and evaluation for the classification and segmentation challenges.
Rules
The VisDA challenge tests adaptation and model transfer, so the rules are different than most challenges. Please read them carefully.
Supervised Training: Teams may only submit test results of models trained on the source domain data and optionally pre-trained on ImageNet. Training on the validation dataset is not allowed for test submissions. Note, this may be different from other challenges. In this year’s VisDA challenge, the goal is to test how well models can adapt from synthetic to real data. Therefore, training on the validation domain is not allowed. To ensure equal comparison, we also do not allow any other external training data, modifying the provided training dataset, or any form of manual data labeling.
Pretraining on ImageNet: If pre-training on the ImageNet ILSVRC classification training data, only the weights can be transferred, not the actual classifiers for specific objects, i.e. participants should not manually exploit correspondences between ImageNet output labels and labels in the data. Please indicate in your method description which pre-trained weights were used for initialization of the model. Teams who place in the top of the “no ImageNet pretraining” track will receive special recognition.
Unsupervised training: Models can be adapted (trained) on the test data in an unsupervised way, i.e. without labels. Adaptation, even unsupervised, on the validation data is not allowed for test submissions. Note, we have released the validation labels to facilitate algorithm development.
Source Models: The performance of a domain adaptation algorithm greatly depends on the baseline performance of the model trained only on source data. We ask that teams submit two sets of results: 1) predictions obtained only with the source-trained model, and 2) predictions obtained with the adapted model. See the development kit for submission formatting details.
Leaderboard:The main leaderboard for each competition track will show results of adapted models and will be used to determine the final team ranks. The expanded leaderboard will additionally show the team's source-only models, i.e. those trained only on the source domain without any adaptation. These results are useful for estimating how much the method improves upon its source-only model, but will not be used to determine team ranks.
FAQ
- Can we train models on data other than the source domain?
- Do we have to use the provided baseline models?
- How many submissions can each team submit per competition track?
- Can multiple teams enter from the same research group?
- Can external data be used?
- Are challenge participants required to reveal all details of their methods?
- Do participants need to adhere to TASK-CV abstract submission deadlines to participate in the challenge?
Participants may elect to pre-train their models only on ImageNet. Please refer to the challenge evaluation instructions found in the DevKit for more details.
No, these are provided for your convenience and are optional.
For the validation domain, the number of submissions per team is limited to 20 upload per day and there are no restrictions on total number of submissions. For the test domain, the number of submissions per team is limited to 1 upload per day and 20 uploads in total. Only one account per team must be used to submit results. Do not create multiple accounts for a single project to circumvent this limit, as this will result in disqualification.
Yes, so long as each team is comprised of different members.
The allowed training data consists of the VisDA 2018 Training set. The VisDA 2018 Validation set can be used to test adaptation to a target domain offline, but cannot be used to train the final submitted model (with or without labels). Optional initialization of models with weights pre-trained on ImageNet is allowed and must be declared in the submission. Please see the challenge rules for more details.
Participants are encouraged but not required to include a brief write-up regarding their methods when submitting their results.
Submission of a TASK-CV workshop abstract is not mandatory to participate in the challenge; however, we request that any teams that wish to be considered for prizes or receive invitation to speak at the workshop submit a 2-page abstract. The top-performing teams that submit abstracts will be invited to present their approaches at the workshop.
Workshop
The challenge is associated with the 5th annual TASK-CV workshop, being held at ECCV 2018 in Munich, Germany. Challenge participants are encouraged to submit abstracts to the main TASK-CV workshop. In order to be considered for any challenge prizes, as well as recieve an invitivation to give a talk about their results at the special VISDA session of the workshop, participants are requested to submit a 2-page abstract directly via email to visda-organizers@googlegroups.com, within 1 week of the challenge end.